Automated Keratoconus Detection
Deep learning model more than 99% accurate, but fails to predict progression.
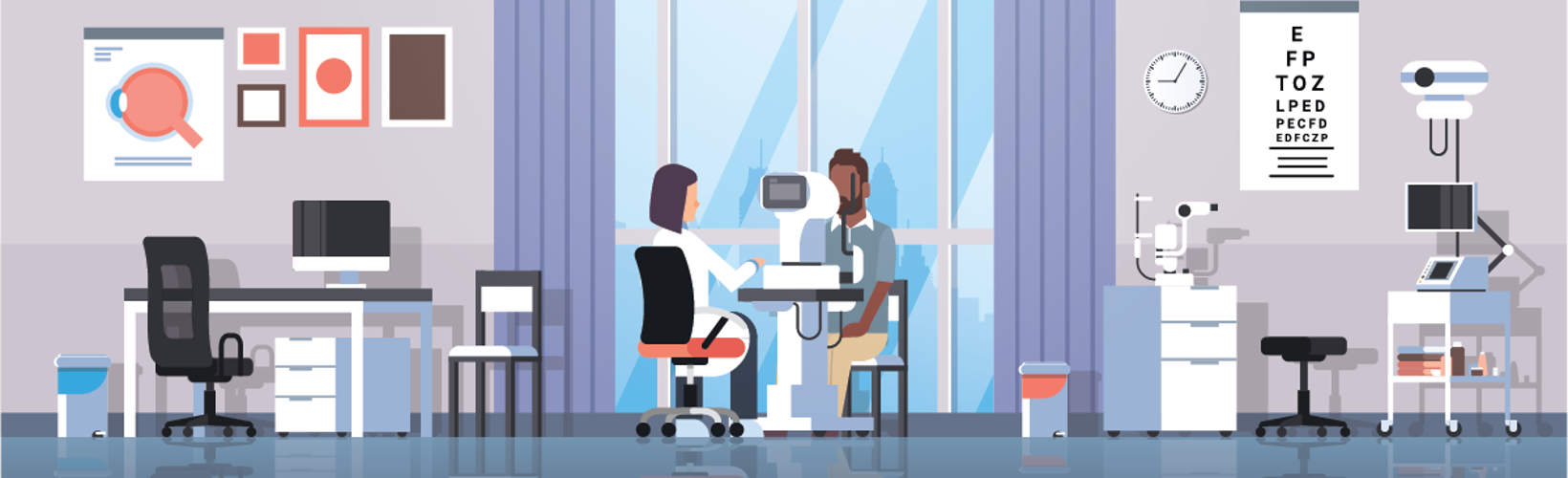
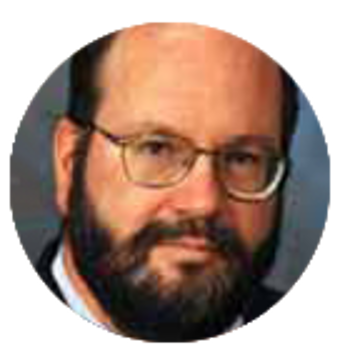
Howard Larkin
Published: Wednesday, June 30, 2021
A deep learning programme using convolutional neural networks (CNN) to assess Pentacam (Oculus) images correctly identified keratoconic and normal corneas more than 99% of the time in a validation test, Henry Liu MD told the Association for Research in Vision and Ophthalmology 2021 Annual Meeting.
The same program displayed accuracy exceeding 87% in staging cases when manifest refraction was added to four Pentacam parameters. However, it was unable to predict progression, possibly due to a limited number of progressing cases to train the system, said Dr Liu, who is a first-year ophthalmology resident at the University of Ottawa, Canada.
TRAINING AND OUTCOMES
Deep learning is a type of machine learning in which a system is exposed to a large set of labelled training images, and develops its own rules for classifying them based on features it detects, rather than having identifying features predetermined by an expert programmer. CNNs act as filters within the deep learning network that identify specific abstract features, progressively increasing accuracy as the image is processed, Dr Liu explained.
Deep learning has been used extensively to automate detection of retinal diseases and glaucoma, but very little research has been done in the field of cornea, Dr Liu noted. This CNN program harnesses widely used Pentacam images, rather than OCT images, for automating keratoconus assessment.
In Dr Liu’s three-stage study with colleagues at Ottawa, a CNN was developed and trained with a dataset of corneal topographies, which included all patients with a diagnosis of keratoconus seen between January 2007 and June 2019, and patients assessed for refractive surgery as controls. A full 80% were used for training and 20% for validation. In all, 2,450 scans, with 1,215 keratoconus and 1,235 controls, were included for stage 1; 985 graded keratoconus scans for stage 2; and 144 keratoconus scans, 72 progressed, and 72 stabilized, for stage 3.
For stage 1 cases, the deep learning model with data from four Pentacam parameters showed an accuracy of 99.3% in discriminating keratoconic from normal corneas. Using individual parameters, anterior elevation, posterior elevation, and anterior curvature yielded accuracy of 98.7%, 98.4%, and 97.6% respectively, with pachymetry maps lagging at 77.1%. For study stage 2, the four parameters yielded 73.5% accuracy grading keratoconus on the four-stage Amsler- Krumeich scale, increasing to 87.8% when manifest refraction was added. For study stage 3, progression was predicted with an accuracy of 53.6%.
“Even though it was not able to predict keratoconus progression, applications of CNNs are encouraging and can be used to solve practical clinical problems, and serve as an adjunct tool in clinical decision-making in the near future,” Dr Liu concluded.
Henry Liu: hliu114@uottawa.ca
Tags: cornea
Latest Articles
Towards a Unified IOL Classification
The new IOL functional classification needs a strong and unified effort from surgeons, societies, and industry.
The 5 Ws of Post-Presbyopic IOL Enhancement
Fine-tuning refractive outcomes to meet patient expectations.
AI Shows Promise for Meibography Grading
Study demonstrates accuracy in detecting abnormalities and subtle changes in meibomian glands.
Are There Differences Between Male and Female Eyes?
TOGA Session panel underlined the need for more studies on gender differences.
Simulating Laser Vision Correction Outcomes
Individualised planning models could reduce ectasia risk and improve outcomes.
Need to Know: Aberrations, Aberrometry, and Aberropia
Understanding the nomenclature and techniques.
Organising for Success
Professional and personal goals drive practice ownership and operational choices.
Update on Astigmatism Analysis
Is Frugal Innovation Possible in Ophthalmology?
Improving access through financially and environmentally sustainable innovation.