Artificial Intelligence
An Open-Eyed View of AI
Reaping the benefits will require good data and careful design and oversight.
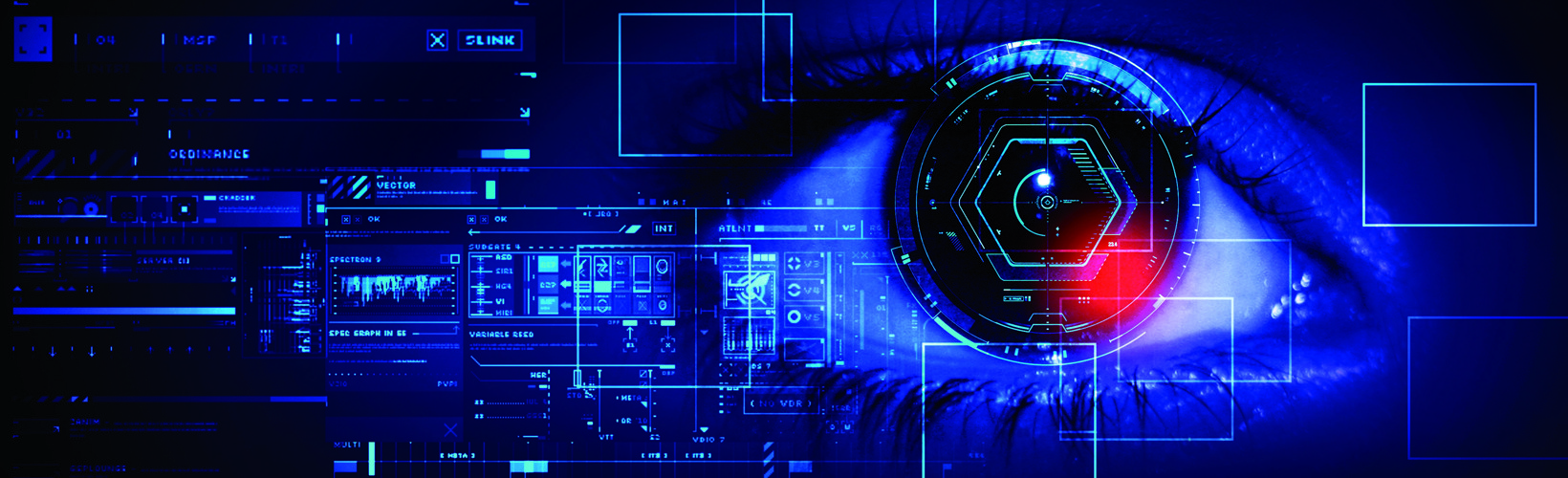
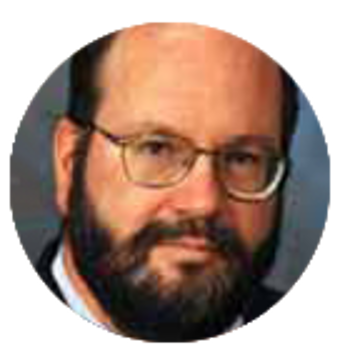
Howard Larkin
Published: Tuesday, August 29, 2023
“ No one is asserting that we should let algorithms treat our patients without oversight. In fact, that’s exactly the opposite. “
“On two occasions I have been asked, ‘Pray, Mr Babbage, if you put into the machine wrong figures, will the right answers come out?’... I am not able rightly to apprehend the kind of confusion of ideas that could provoke such a question.” – Charles Babbage, inventor of the digital programmable computer, from Life of a Philosopher (London, 1864).
In 1837, Charles Babbage designed a programmable mechanical calculating device that is the conceptual model for most electronic digital computers today. With the above quote, he also articulated a computer truism later formulated as “GIGO”—garbage in, garbage out. In other words, the quality of any computer output depends on the quality of the data and programming going in.
And so it remains, even in the realm of artificial intelligence (AI). AI is any computer application that does something normally thought to require human intelligence, Dr Pearse A Keane, an ophthalmologist and professor of artificial medical intelligence, told EuroTimes. However, AI deep learning models go beyond traditional computer programs by independently identifying relationships among data points not prespecified by programmers. Further, they can be programmed to learn and adjust their algorithms based on new information. This gives AI enormous and unforeseeable transformational potential on par with the advent of personal computers—and ophthalmology is at the forefront, he said.
But whether they are narrowly focused, supervised applications trained on labelled ocular images or other curated data sets, or unsupervised or generative applications powered by large language models (LLM) accessing hundreds of billions of words, images, recordings, or medical records, AI-enabled programs are still computer programs. As such, they require careful design, testing, training, and ongoing supervision to work reliably and accurately— at least for now.
“We have seen a lot of hype around AI. The interesting and scary thing is progress is accelerating—even in the last few months—that is really blowing everyone’s mind outside healthcare. It will be absolutely huge,” Dr Keane said. “But we need to balance enthusiasm with caution for anything used in healthcare.”
So, just as ophthalmologists wouldn’t send a referral note or answer a patient question using only what the electronic medical record provides or diagnose glaucoma progression based on automated visual field analysis alone, the output of any AI system, no matter how sophisticated, always should be carefully evaluated and edited, said Dr Ranya Habash, co-chair of AI for the American- European Congress of Ophthalmic Surgery.
“No one is asserting that we should let algorithms treat our patients without oversight. In fact, that’s exactly the opposite,” Dr Habash said. “We can allow AI to perform the tedious tasks to help us be more efficient; then, we oversee the output to make sure things are accurate before they go out. It’s our responsibility and an obligation.”
Model drift and hallucinations
There are many types of AI, but those used in healthcare are predominantly deep learning variants of machine learning. They use neural networks to iteratively identify, examine, and statistically test correlations among data points based on images or other digital data such as biometry measurements or text.
The goal is to develop models capable of predicting likely diagnoses or outcomes in patients outside the training data set used, such as screening for diabetic retinopathy or selecting the appropriate IOL power for cataract surgery. LLMs may also help draft documentation, patient communication, surgical plans, reports, and papers, or even assist in resolving diagnostic dilemmas.
Because these statistical models are empirical, their predictive power depends heavily on the make-up of their data training sets. In general, the larger and more representative the training set is of the general patient population, the more accurate the model will be for clinical use.
Typically, only part of the sample data set trains the models, with the resulting algorithm tested for accuracy on the remaining portion. Tweaking and rerunning the model occurs at this stage. But before its use in practice, it should also be clinically validated with other methods, Dr Keane said.
Testing for approved AI medical devices is stringent, and devices in clinical use should meet that standard, he added. AI-trained devices currently approved by the US Food and Drug Administration (FDA) are locked, meaning they do not learn, and the model doesn’t change. The agency is developing regulations to accommodate machine learning by requiring a prospective plan to revise and test models without further approval.
Data quality is also critical, Dr Mark Lobanoff told EuroTimes. But clinical data gathered from large groups of practices can be unreliable due to differences in when and how it is collected, even the calibration of test equipment.
In his work with Bausch + Lomb developing AI applications for eyeTELLIGENCE, an ophthalmology software platform, Dr Lobanoff addresses the issue by using a subgroup of data known to be meticulously collected. Models, such as those calculating IOL power or detecting glaucoma progression, are developed using this set and then tested in the larger, less curated data set to find tweaks to improve performance.
Clinical validation involves running beta versions alongside existing methods and comparing the outcomes the models predicted with those achieved using the existing methods. Only then will the AI models be ready for clinical use, which Dr Lobanoff said is about two years off for eyeTELLIGENCE.
Dr Lobanoff said care also must be taken using generative LLMs, such as ChatGPT. “We don’t always really understand what AI is doing, how it finds a solution.” This can lead to “hallucinations”—or a confident presentation of wildly wrong information. Generative AI systems making up references or describing how the Golden Gate Bridge moved to Egypt are examples. LLMs are being revised to reduce or eliminate these problems. But he advised always checking the text for accuracy before signing off on it.
The clinician’s role
While most clinicians will not participate directly in developing AI applications, these tools will likely become ubiquitous soon, Dr Keane said. Practising clinicians will not only use such applications— many will likely provide clinical data for updating them through electronic systems.
As AI applications become available, clinicians need to educate themselves on appropriate use, Dr Keane said. “Learn how to identify their strengths and weaknesses. In a certain type of patient, an algorithm might not be so accurate—that is the kind of learning we will need.” For example, an algorithm developed on average axial lengths may not be as accurate as one developed specifically for shorter eyes.
Awareness of the importance of collecting accurate data is also critical, Dr Lobanoff said, noting precise postoperative manifest refractions are particularly needed to evaluate cataract procedures. This could drive culture changes in practices to collect such information more regularly and rigorously, benefitting these practices with better AI models. “Accuracy means a happier patient.”
Pearse A Keane MD is an ophthalmologist at Moorfields Eye Hospital, London, UK, and professor of artificial medical intelligence at Univ ersity College London. p.keane@ucl.ac.uk
Ranya Habash MD is an ophthalmologist and assistant professor of ophthalmology at Bascom Palmer Eye Institute, Miami, US. ranya@habash.net
Mark Lobanoff MD is an ophthalmologist and founder and president of OVO LASIK + LENS, a private clinic; founder and CEO of Phorcides, a LASIK software firm. mlobanoff@gmail.com
Latest Articles
Simulators Benefit Surgeons and Patients
Helping young surgeons build confidence and expertise.
Making Female Leadership More than a Moment
A remarkable global confluence of women in key positions.
ESCRS Talks Technology at AAO
Europe adopts technological advances, US still waiting for lenses and lasers.
Sorting Out Simultaneous Vision IOLs
The ESCRS Eye Journal Club discuss a new landmark paper on IOL classification and the need for harmonisation of terminology for presbyopic IOLs.
Big Advantages to Small-Aperture IOLs
Small-aperture IOLs offer superior image quality with increased range of focus.
Prioritising Self-Care
Benefits of maintaining physical, emotional, and mental health extend beyond the personal sphere.
Valuing Clinical Trial Design
How inclusivity and diversity can enhance scientific accuracy in research.
Knowing Iris Repair: Using Iridodiathermy in Iris Surgery
Prepare for decentred pupils and uneven irides in multiple situations.
Neuroprotectant Treatment for MacTel Type 2
Intravitreal implant releasing ciliary neurotrophic factor found safe and effective in pivotal trials.